HMC - Heterogeneous Model Compilers for Uncertain Environments
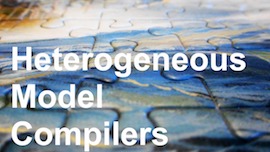
The overall goal of this project is to develop new techniques and tools that make it easier to develop complex systems, by combining heterogeneous models and to automatically generate efficient runtime systems on heterogeneous platforms. The concrete expected output is an open-source model-based compiler that will be directly usable to the industry.
Introduction
The extensive use of information communication technology in our society has drastically changed the way we live of lives. Complex systems, such as modern cars, satellites, aircraft, medical devices, and wireless telecommunication systems constantly improve their performance and utility. To cope with constantly growing systems complexities, model-based design and machine learning have emerged as two essential design and implementation techniques. Model-based design methods enable virtual prototyping, verification, and simulation prior building real systems. Machine learning techniques can perform probabilistic reasoning, learn from data, reason under uncertainty, and enable system innovations that were not possible before. Yet these techniques have not reached close to their real potentials.The problem
Today's rapid progress is impeded by the learning curve and incompatibility between the tremendous amounts of existing modeling formalisms, tools, and machine learning algorithms. We do not know how to modularize and compose these formalisms and algorithms together in a systematic, simple, sound, and efficient way. This heterogeneous landscape also include the target platforms: different specialized tools are needed for compiling to various heterogeneous targets, varying from distributed cluster computing platforms to specialized real-time systems. This semantic gap between high level modeling abstractions and efficient implementations is one of the main obstacles from a more wide spread use of the combination of high-level modeling and machine learning.Research themes
The project can divided into three different research themes, as depicted in the Figure below. Each theme has its own research goal:- Research theme 1: Heterogeneous model semantics
Goal: develop a new foundation for heterogeneous model semantics, where equation-based models and computational models can, in a sound manner, be composed with probabilistic models. - Research theme 2: Modular self-learning model compiler
Goal: design and implement an open source modular self-learning model compiler that can combine, select, and specialize algorithms (machine learning inference and mixed symbolic/numerical solvers) for a heterogeneous set of model inputs. - Research theme 3: Optimization for heterogeneous target platforms
Goal: construct methods and algorithms for automatic code generation and optimization for heterogeneous target platforms, including cluster computing platforms for offline big data computing, and real-time platforms with timing constraints for online real-time decision problems.
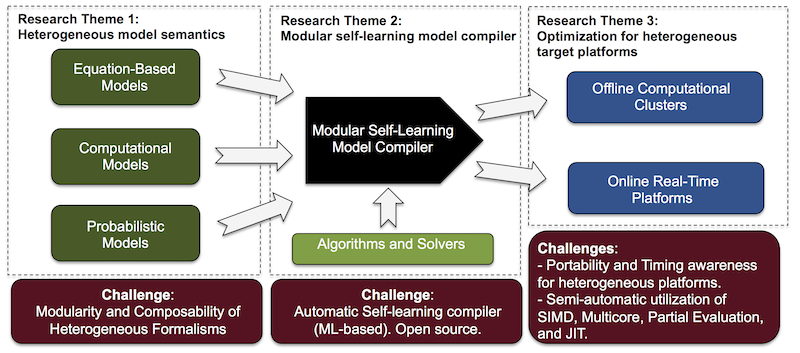
Collaboration with industry
To impose concrete evidence for the relevance and importance of our research to the Swedish industry, we have identified three concrete applications. The application demonstrators are: i) energy improvement and anomaly detection in telecommunication systems (with Ericsson), ii) automation of container ports (with ABB), and system testing of CubeSat satellites (with KTH Space Center).
This project is financially supported by the Swedish Foundation for Strategic Research (SSF).
This is a personal web page. More information.