EDAREM
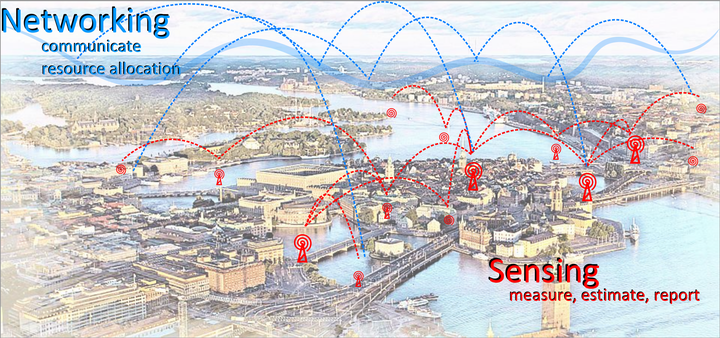
Efficient Data Representation and Machine Learning over Next Generation Networks, Principal Investigator, WASP Autonomous Systems and Artificial Intelligence Sweden, 2020-2024 (1 M€). In the recent years, the outstanding progress of information technologies is marked by the advent of various machine learning (ML) techniques and the subsequent rise of artificial intelligence (AI). This has revolutionized the research and development landscape in related disciplines at a large scale with numerous successful applications. In contrast to the so-called rule-based methods, ML is grounded in the principle of statistical learning, where sufficiently informative measured data train highly versatile models. The recent success of ML methods hinges on the advances on computation technology in combination with the unique opportunity to access an unprecedented amount of data, achieved by the growth of relatively low-cost information-based monitoring and controlling apparatus in real-world applications. In the ML literature, despite a limited number of highly popular benchmark examples such as MNIST, CIFAR, ImageNet1, such an extensive amount of data and the required computation is widely available by only resorting to a network of various sensory and computational elements. The communication networks among such elements are a vital element of intelligence in realistic scenarios such as those offered by Internet of Things (IoT) and upcoming 5G wireless networks. The indispensable role of communication for the success of AI in such networks has recently gained an increased attention. Yet, existing ML method over networks are mostly intended for data centers and thus assume highly performing and proprietary communication networks, whereas IoT and 5G networks have substantially different characteristics. A rigorous mathematical framework for ML over communication networks is still lacking to a great extent. Accordingly, the purpose of this research project is to contribute to the development of the theoretical foundations ML over future communication networks. Beside providing the capacity for harvesting a massive amount of data, such networks will significantly reduce the run time by dividing the computational burden among multiple computational agents, being highly favorable for time-dependent and adaptive applications.