[ED.
SPECULATIONS
ON THE FUTURE OF SCIENCE
By Kevin Kelly
Science
will continue to surprise us with what it discovers and creates;
then it will astound us by devising new methods to surprises
us. At the core of science's self-modification is technology.
New tools enable new structures of knowledge and new ways of
discovery. The achievement of science is to know new things;
the evolution of science is to know them in new ways. What evolves
is less the body of what we know and more the nature of our knowing
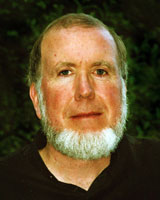
Introduction
by Stewart Brand
Science,
says Kevin Kelly, is the process of changing how we know things. It
is the foundation our culture and society. While civilizations
come and go, science grows steadily onward. It does this by
watching itself.
Recursion
is the essence of science. For example, science papers cite
other science papers, and that process of research pointing at itself
invokes a whole higher level, the emergent shape of citation space. Recursion
always does that. It is the engine of scientific progress and
thus of the progress of society.
A particularly
fruitful way to look at the history of science is to study how science
itself has changed over time, with an eye to what that trajectory
might suggest about the future. Kelly chronicled a sequence
of new recursive devices in science...
2000 BC — First
text indexes
200 BC — Cataloged library (at Alexandria)
1000 AD — Collaborative encyclopedia
1590 — Controlled experiment (Roger Bacon)
1600 — Laboratory
1609 — Telescopes and microscopes
1650 — Society of experts
1665 — Repeatability (Robert Boyle)
1665 — Scholarly journals
1675 — Peer review
1687 — Hypothesis/prediction (Isaac Newton)
1920 — Falsifiability (Karl Popper)
1926 — Randomized design (Ronald Fisher)
1937 — Controlled placebo
1946 — Computer simulation
1950 — Double blind experiment
1962 — Study of scientific method (Thomas Kuhn)
Projecting
forward, Kelly had five things to say about the next 100 years in
science...
1) There
will be more change in the next 50 years of science than in the last
400 years.
2) This
will be a century of biology. It is the domain with the most
scientists, the most new results, the most economic value, the most
ethical importance, and the most to learn.
3) Computers
will keep leading to new ways of science. Information is growing
by 66% per year while physical production grows by only 7% per year. The
data volume is growing to such levels of "zillionics" that
we can expect science to compile vast combinatorial libraries, to
run combinatorial sweeps through possibility space (as Stephen Wolfram
has done with cellular automata), and to run multiple competing hypotheses
in a matrix. Deep realtime simulations and hypothesis search
will drive data collection in the real world.
4) New
ways of knowing will emerge. "Wikiscience" is leading
to perpetually refined papers with a thousand authors. Distributed
instrumentation and experiment, thanks to miniscule transaction cost,
will yield smart-mob, hive-mind science operating "fast, cheap, & out
of control." Negative results will have positive value
(there is already a "Journal of Negative Results in Biomedicine").
Triple-blind experiments will emerge through massive non-invasive
statistical data collection--- no one, not the subjects or the experimenters,
will realize an experiment was going on until later. (In the Q&A,
one questioner predicted the coming of the zero-author paper, generated
wholly by computers.)
5) Science
will create new levels of meaning. The Internet already is
made of one quintillion transistors, a trillion links, a million
emails per second, 20 exabytes of memory. It is approaching
the level of the human brain and is doubling every year, while the
brain is not. It is all becoming effectively one machine. And
we are the machine.
"Science
is the way we surprise God," said Kelly. "That's
what we're here for." Our moral obligation is to generate
possibilities, to discover the infinite ways, however complex and
high-dimension, to play the infinite game. It will take all
possible species of intelligence in order for the universe to understand
itself. Science, in this way, is holy. It is a divine trip.
— Stewart
Brand
KEVIN
KELLY helped launch Wired magazine in 1993, and served as
its Executive Editor until January 1999. He is now Editor-At-Large
for WiredFrom 1984 to 1990 Kelly was publisher and editor
of the Whole Earth Review. In the late 80s, Kelly conceived
and oversaw the publication of four versions of the Whole Earth
Catalogs. He was a founding board member of the WELL.
Kelly
is the author of Out of Control and New Rules for the
New Economy, and his writing has appeared in many national and
international publications such as the New York Times, The Economist,
Time, Harpers, Science, GQ, and Esquire.
Kevin
Kelly's Edge Bio page
SPECULATIONS
ON THE FUTURE OF SCIENCE
(KEVIN
KELLY:) Science
will continue to surprise us with what it discovers and creates;
then it will astound us by devising new methods to surprises us.
At the core of science's self-modification is technology. New tools
enable new structures of knowledge and new ways of discovery. The
achievement of science is to know new things; the evolution of science
is to know them in new ways. What evolves is less the body of what
we know and more the nature of our knowing.
Technology
is, in its essence, new ways of thinking. The most powerful type
of technology, sometimes called enabling technology, is a thought
incarnate which enables new knowledge to find and develop news ways
to know. This kind of recursive bootstrapping is how science evolves.
As in every type of knowledge, it accrues layers of self-reference
to its former state.
New informational
organizations are layered upon the old without displacement, just
as in biological evolution. Our brains are good examples. We retain
reptilian reflexes deep in our minds (fight or flight) while the
more complex structuring of knowledge (how to do statistics) is layered
over those primitive networks. In the same way, older methods of
knowing (older scientific methods) are not jettisoned; they are simply
subsumed by new levels of order and complexity. But the new tools
of observation and measurement, and the new technologies of knowing,
will alter the character of science, even while it retains the old
methods.
I'm willing
to bet the scientific method 400 years from now will differ from
today's understanding of science more than today's science method
differs from the proto-science used 400 years ago. A sensible forecast
of technological innovations in the next 400 years is beyond our
imaginations (or at least mine), but we can fruitfully envision technological
changes that might occur in the next 50 years.
Based
on the suggestions of the observers above, and my own active imagination,
I offer the following as possible near-term advances in the evolution
of the scientific method.
Compiled
Negative Results — Negative results are saved, shared, compiled
and analyzed, instead of being dumped. Positive results may increase
their credibility when linked to negative results. We already have
hints of this in the recent decision of biochemical journals to require
investigators to register early phase 1 clinical trials. Usually
phase 1 trials of a drug end in failure and their negative results
are not reported. As a public heath measure, these negative results
should be shared. Major journals have pledged not to publish the
findings of phase 3 trials if their earlier phase 1 results had not
been reported, whether negative or not.
Triple
Blind Experiments – In a double blind experiment neither researcher
nor subject are aware of the controls, but both are aware of the
experiment. In a triple blind experiment all participants are blind
to the controls and to the very fact of the experiment itself. The
way of science depends on cheap non-invasive sensor running continuously
for years generating immense streams of data. While ordinary life
continues for the subjects, massive amounts of constant data about
their lifestyles are drawn and archived. Out of this huge database,
specific controls, measurements and variables can be "isolated" afterwards.
For instance, the vital signs and lifestyle metrics of a hundred
thousand people might be recorded in dozens of different ways for
20-years, and then later analysis could find certain variables (smoking
habits, heart conditions) and certain ways of measuring that would
permit the entire 20 years to be viewed as an experiment – one
that no one knew was even going on at the time. This post-hoc analysis
depends on pattern recognition abilities of supercomputers. It removes
one more variable (knowledge of experiment) and permits greater freedom
in devising experiments from the indiscriminate data.
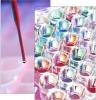
Combinatorial
Sweep Exploration – Much of the unknown can be explored by
systematically creating random varieties of it at a large scale.
You can explore the composition of ceramics (or thin films, or rare-earth
conductors) by creating all possible types of ceramic (or thin films,
or rare-earth conductors), and then testing them in their millions.
You can explore certain realms of proteins by generating all possible
variations of that type of protein and they seeing if they bind to
a desired disease-specific site. You can discover new algorithms
by automatically generating all possible programs and then running
them against the desired problem. Indeed all possible Xs of almost
any sort can be summoned and examined as a way to study X. None of
this combinatorial exploration was even thinkable before robotics
and computers; now both of these technologies permit this brute force
style of science. The parameters of the emergent "library" of
possibilities yielded by the sweep become the experiment. With sufficient
computational power, together with a pool of proper primitive parts,
vast territories unknown to science can be probed in this manner.
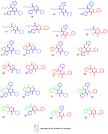
Evolutionary
Search – A combinatorial exploration can be taken even further.
If new libraries of variations can be derived from the best of a
previous generation of good results, it is possible to evolve solutions.
The best results are mutated and bred toward better results. The
best testing protein is mutated randomly in thousands of way, and
the best of that bunch kept and mutated further, until a lineage
of proteins, each one more suited to the task than its ancestors,
finally leads to one that works perfectly. This method can be applied
to computer programs and even to the generation of better hypothesis.
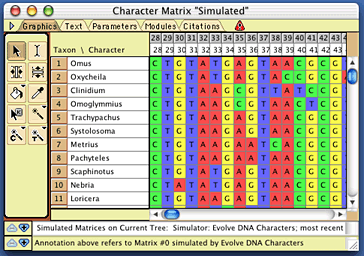
Multiple
Hypothesis Matrix – Instead of proposing a series of single
hypothesis, in which each hypothesis is falsified and discarded until
one theory finally passes and is verified, a matrix of many hypothesis
scenarios are proposed and managed simultaneously. An experiment
travels through the matrix of multiple hypothesis, some of which
are partially right and partially wrong. Veracity is statistical;
more than one thesis is permitted to stand with partial results.
Just as data were assigned a margin of error, so too will hypothesis.
An explanation may be stated as: 20% is explained by this theory,
35% by this theory, and 65% by this theory. A matrix also permits
experiments with more variables and more complexity than before.
Pattern
Augmentation – Pattern-seeking software which recognizes a
pattern in noisy results. In large bodies of information with many
variables, algorithmic discovery of patterns will become necessary
and common. These exist in specialized niches of knowledge (such
particle smashing) but more general rules and general-purpose pattern
engines will enable pattern-seeking tools to become part of all data
treatment.
Adaptive
Real Time Experiments – Results evaluated, and large-scale
experiments modified in real time. What we have now is primarily
batch-mode science. Traditionally, the experiment starts, the results
are collected, and then conclusions reached. After a pause the next
experiment is designed in response, and then launched. In adaptive
experiments, the analysis happens in parallel with collection, and
the intent and design of the test is shifted on the fly. Some medical
tests are already stopped or re-evaluated on the basis of early findings;
this method would extend that method to other realms. Proper methods
would be needed to keep the adaptive experiment objective.
AI Proofs – Artificial
intelligence will derive and check the logic of an experiment. Ever
more sophisticated and complicated science experiments become ever
more difficult to judge. Artificial expert systems will at first
evaluate the scientific logic of a paper to ensure the architecture
of the argument is valid. It will also ensure it publishes the required
types of data. This "proof review" will augment the peer-review
of editors and reviewers. Over time, as the protocols for an AI check
became standard, AI can score papers and proposals for experiments
for certain consistencies and structure. This metric can then be
used to categorize experiments, to suggest improvements and further
research, and to facilitate comparisons and meta-analysis. A better
way to inspect, measure and grade the structure of experiments would
also help develop better kinds of experiments.
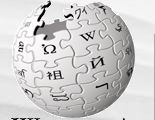
Wiki-Science – The
average number of authors per paper continues to rise. With massive
collaborations, the numbers will boom. Experiments involving thousands
of investigators collaborating on a "paper" will commonplace.
The paper is ongoing, and never finished. It becomes a trail of edits
and experiments posted in real time — an ever evolving "document."
Contributions are not assigned. Tools for tracking credit and contributions
will be vital. Responsibilities for errors will be hard to pin down.
Wiki-science will often be the first word on a new area. Some researchers
will specialize in refining ideas first proposed by wiki-science.
Defined
Benefit Funding — Ordinarily science is funded by the experiment
(results not guaranteed) or by the investigator (nothing guaranteed).
The use of prize money for particular scientific achievements will
play greater roles. A goal is defined, funding secured for the first
to reach it, and the contest opened to all. The Turing Test prize
awarded to the first computer to pass the Turing Test as a passable
intelligence. Defined Benefit Funding can also be combined with prediction
markets, which set up a marketplace of bets on possible innovations.
The bet winnings can encourage funding of specific technologies.
Zillionics – Ubiquitous
always-on sensors in bodies and environment will transform medical,
environmental, and space sciences. Unrelenting rivers of sensory
data will flow day and night from zillions of sources. The exploding
number of new, cheap, wireless, and novel sensing tools will require
new types of programs to distill, index and archive this ocean of
data, as well as to find meaningful signals in it. The field of "zillionics" — -
dealing with zillions of data flows — - will be essential in
health, natural sciences, and astronomy. This trend will require
further innovations in statistics, math, visualizations, and computer
science. More is different. Zillionics requires a new scientific
perspective in terms of permissible errors, numbers of unknowns,
probable causes, repeatability, and significant signals.
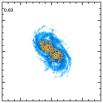
Deep Simulations – As
our knowledge of complex systems advances, we can construct more
complex simulations of them. Both the success and failures of these
simulations will help us to acquire more knowledge of the systems.
Developing a robust simulation will become a fundamental part of
science in every field. Indeed the science of making viable simulations
will become its own specialty, with a set of best practices, and
an emerging theory of simulations. And just as we now expect a hypothesis
to be subjected to the discipline of being stated in mathematical
equations, in the future we will expect all hypothesis to be exercised
in a simulation. There will also be the craft of taking things known
only in simulation and testing them in other simulations—sort
of a simulation of a simulation.
Hyper-analysis
Mapping – Just as meta-analysis gathered diverse experiments
on one subject and integrated their (sometimes contradictory) results
into a large meta-view, hyper-analysis creates an extremely large-scale
view by pulling together meta-analysis. The cross-links of references,
assumptions, evidence and results are unraveled by computation, and
then reviewed at a larger scale which may include data and studies
adjacent but not core to the subject. Hyper-mapping tallies not only
what is known in a particular wide field, but also emphasizes unknowns
and contradictions based on what is known outside that field. It
is used to integrate a meta-analysis with other meta-results, and
to spotlight "white spaces" where additional research would
be most productive.
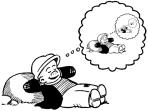
Return
of the Subjective – Science came into its own when it managed
to refuse the subjective and embrace the objective. The repeatability
of an experiment by another, perhaps less enthusiastic, observer
was instrumental in keeping science rational. But as science plunges
into the outer limits of scale – at the largest and smallest
ends – and confronts the weirdness of the fundamental principles
of matter/energy/information such as that inherent in quantum effects,
it may not be able to ignore the role of observer. Existence seems
to be a paradox of self-causality, and any science exploring the
origins of existence will eventually have to embrace the subjective,
without become irrational. The tools for managing paradox are still
undeveloped.